6 Joining Data with dplyr
https://learn.datacamp.com/courses/joining-data-with-dplyr
Main functions and concepts covered in this BP chapter:
- The
inner_join
verb- Joining parts and part categories
- Joining with a one-to-many relationship
- Joining parts and inventories
- Joining in either direction
- Joining three or more tables
- Joining three tables
- What’s the most common color?
- The
left_join
verb- Left joining two sets by part and color
- Left joining two sets by color
- Finding an observation that doesn’t have a match
- The
right_join
verb- Counting part colors
- Cleaning up your count
- Joining tables to themselves
- Joining themes to their children
- Joining themes to their grandchildren
- Left joining a table to itself
- The
full_join
verb- Differences between Batman and Star Wars
- Aggregating each theme
- Full joining Batman and Star Wars LEGO parts
- Comparing Batman and Star Wars LEGO parts
- The
semi_join
andanti_join
verbs- Something within one set but not another
- What colors are included in at least one set?
- Which set is missing version 1?
- Visualizing set differences
- Aggregating sets to look at their differences
- Combining sets
- Visualizing the difference: Batman and Star Wars
- Stack Overflow questions
- Left joining questions and tags
- Comparing scores across tags
- What tags never appear on R questions?
- Joining questions and answers
- Finding gaps between questions and answers
- Joining question and answer counts
- Joining questions, answers, and tags
- Average answers by question
- The
bind_rows
verb- Joining questions and answers with tags
- Binding and counting posts with tags
- Visualizing questions and answers in tags
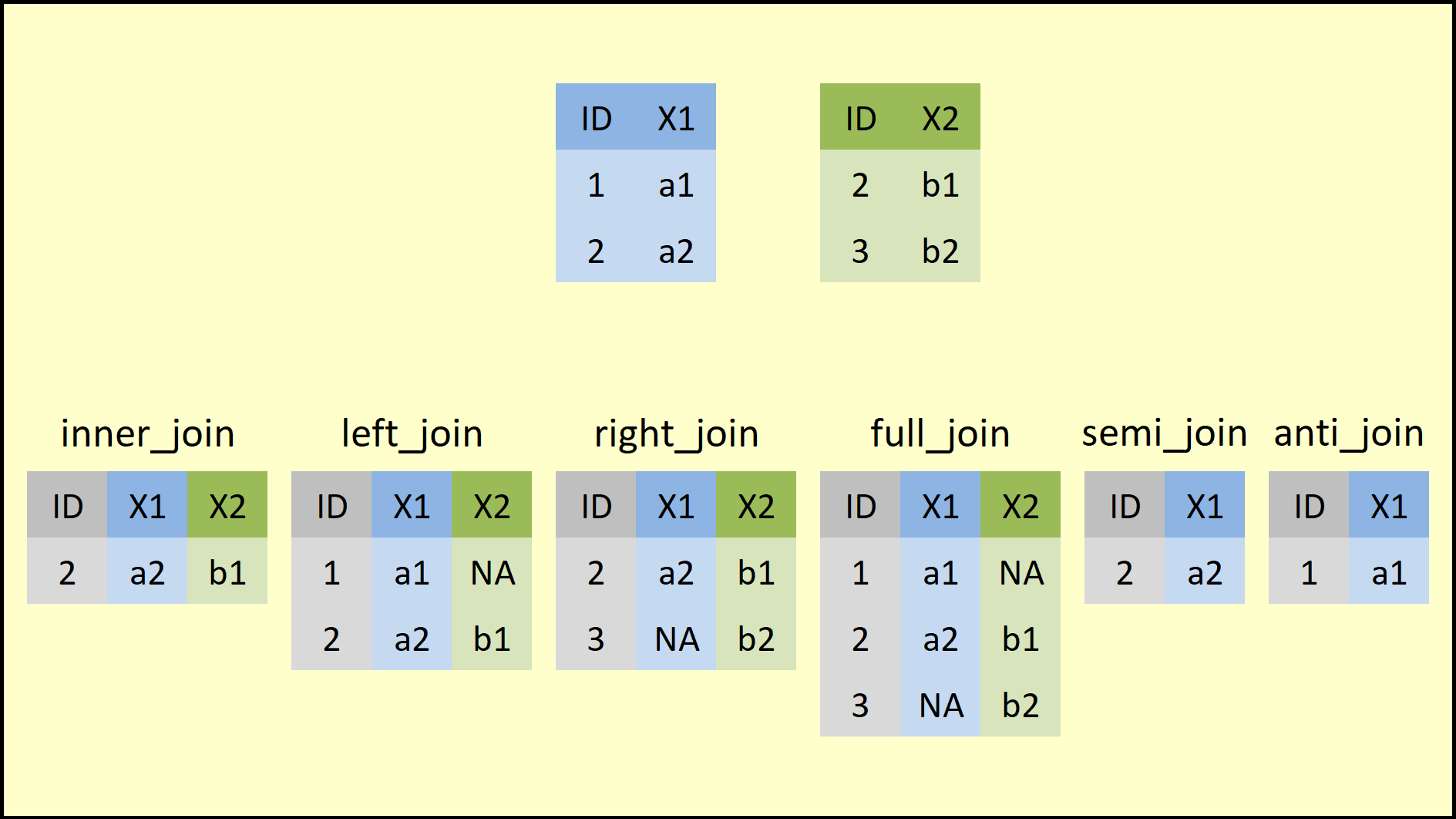
source: https://statisticsglobe.com/r-dplyr-join-inner-left-right-full-semi-anti
Packages used in this chapter:
## Load all packages used in this chapter
library(tidyverse) #includes dplyr, ggplot2, and other common packages
## ── Attaching core tidyverse packages ──────────────────────── tidyverse 2.0.0 ──
## ✔ dplyr 1.1.4 ✔ readr 2.1.4
## ✔ forcats 1.0.0 ✔ stringr 1.5.1
## ✔ ggplot2 3.4.4 ✔ tibble 3.2.1
## ✔ lubridate 1.9.3 ✔ tidyr 1.3.0
## ✔ purrr 1.0.2
## ── Conflicts ────────────────────────────────────────── tidyverse_conflicts() ──
## ✖ dplyr::filter() masks stats::filter()
## ✖ dplyr::lag() masks stats::lag()
## ℹ Use the conflicted package (<http://conflicted.r-lib.org/>) to force all conflicts to become errors
Datasets used in this chapter:
## Load datasets used in this chapter
parts <- read_rds("data/parts.rds")
part_categories <- read_rds("data/part_categories.rds")
inventory_parts <- read_rds("data/inventory_parts.rds")
inventories <- read_rds("data/inventories.rds")
sets <- read_rds("data/sets.rds")
colors <- read_rds("data/colors.rds")
themes <- read_rds("data/themes.rds")
questions <- read_rds("data/questions.rds")
question_tags <- read_rds("data/question_tags.rds")
tags <- read_rds("data/tags.rds")
answers <- read_rds("data/answers.rds")
Tip: one of the common mistakes that leads to people getting stuck in the by
argument, mixing up by=c("var1"="var2")
versus by=c("var1", "var2")
Note: In some exercises they have you replace NAs with 0. This is correct in these particular cases, but this is not always correct. It’s only correct if NA actually represents 0 (which it does in these exercises). (For example, if we had a dataset on people that asked how many cigarettes smoked per day and it was NA for some observations, we couldn’t assume NA means 0 because it might actually be 40 but they just didn’t answer that question.)
6.1 Joining Tables
6.1.1 The inner_join verb
When trying to join two tables, we’ll need to join the two tables. To do this, you use inner join. The inner_join
is the key to bring tables together. To use it, you need to provide the two tables that must be joined and the columns on which they should be joined.
6.1.1.1 Joining parts and part categories
In this exercise, you’ll join a list of LEGO parts, available as parts
, with these parts’ corresponding categories, available as part_categories
. For example, the part Sticker Sheet 1 for Set 1650-1
is from the Stickers
part category. You can join these tables to see all parts’ categories!
Add the correct joining verb, the name of the second table, and the joining column for the second table.
# Add the correct verb, table, and joining column
parts %>%
inner_join(part_categories, by = c("part_cat_id" = "id"))
## # A tibble: 17,501 × 4
## part_num name.x part_cat_id name.y
## <chr> <chr> <dbl> <chr>
## 1 0901 Baseplate 16 x 30 with Set 080 Yellow House Pr… 1 Basep…
## 2 0902 Baseplate 16 x 24 with Set 080 Small White Hou… 1 Basep…
## 3 0903 Baseplate 16 x 24 with Set 080 Red House Print 1 Basep…
## 4 0904 Baseplate 16 x 24 with Set 080 Large White Hou… 1 Basep…
## 5 1 Homemaker Bookcase 2 x 4 x 4 7 Conta…
## 6 10016414 Sticker Sheet #1 for 41055-1 58 Stick…
## 7 10026stk01 Sticker for Set 10026 - (44942/4184185) 58 Stick…
## 8 10039 Pullback Motor 8 x 4 x 2/3 44 Mecha…
## 9 10048 Minifig Hair Tousled 65 Minif…
## 10 10049 Minifig Shield Broad with Spiked Bottom and Cu… 27 Minif…
## # ℹ 17,491 more rows
Now, use the suffix argument to add “_part” and “_category” suffixes to replace the name.x and name.y fields.
# Use the suffix argument to replace .x and .y suffixes
parts %>%
inner_join(part_categories, by = c("part_cat_id" = "id"), suffix = c("_part", "_category"))
## # A tibble: 17,501 × 4
## part_num name_part part_cat_id name_category
## <chr> <chr> <dbl> <chr>
## 1 0901 Baseplate 16 x 30 with Set 080 Yellow H… 1 Baseplates
## 2 0902 Baseplate 16 x 24 with Set 080 Small Wh… 1 Baseplates
## 3 0903 Baseplate 16 x 24 with Set 080 Red Hous… 1 Baseplates
## 4 0904 Baseplate 16 x 24 with Set 080 Large Wh… 1 Baseplates
## 5 1 Homemaker Bookcase 2 x 4 x 4 7 Containers
## 6 10016414 Sticker Sheet #1 for 41055-1 58 Stickers
## 7 10026stk01 Sticker for Set 10026 - (44942/4184185) 58 Stickers
## 8 10039 Pullback Motor 8 x 4 x 2/3 44 Mechanical
## 9 10048 Minifig Hair Tousled 65 Minifig Head…
## 10 10049 Minifig Shield Broad with Spiked Bottom… 27 Minifig Acce…
## # ℹ 17,491 more rows
6.1.2 Joining with a one-to-many relationship
In the first lesson, you joined the sets table to the themes table. But not all joins work that way.
6.1.2.1 Joining parts and inventories
The LEGO data has many tables that can be joined together. Often times, some of the things you care about may be a few tables away (we’ll get to that later in the course). For now, we know that parts
is a list of all LEGO parts, and a new table, inventory_parts
, has some additional information about those parts, such as the color_id
of each part you would find in a specific LEGO kit.
Let’s join these two tables together to observe how joining parts
with inventory_parts
increases the size of your table because of the one-to-many relationship that exists between these two tables.
Connect the parts and inventory_parts
tables by their part numbers (part_num
) using an inner join.
# Combine the parts and inventory_parts tables
parts %>%
inner_join(inventory_parts, by = "part_num")
## # A tibble: 258,958 × 6
## part_num name part_cat_id inventory_id color_id quantity
## <chr> <chr> <dbl> <dbl> <dbl> <dbl>
## 1 0901 Baseplate 16 x 30 with S… 1 1973 2 1
## 2 0902 Baseplate 16 x 24 with S… 1 1973 2 1
## 3 0903 Baseplate 16 x 24 with S… 1 1973 2 1
## 4 0904 Baseplate 16 x 24 with S… 1 1973 2 1
## 5 1 Homemaker Bookcase 2 x 4… 7 508 15 1
## 6 1 Homemaker Bookcase 2 x 4… 7 1158 15 2
## 7 1 Homemaker Bookcase 2 x 4… 7 6590 15 2
## 8 1 Homemaker Bookcase 2 x 4… 7 9679 15 2
## 9 1 Homemaker Bookcase 2 x 4… 7 12256 1 2
## 10 1 Homemaker Bookcase 2 x 4… 7 13356 15 1
## # ℹ 258,948 more rows
6.1.2.2 Joining in either direction
An inner_join
works the same way with either table in either position. The table that is specified first is arbitrary, since you will end up with the same information in the resulting table either way.
Let’s prove this by joining the same two tables from the last exercise in the opposite order!
Connect the inventory_parts
and parts
tables by their part numbers (part_num
) using an inner join.
# Combine the parts and inventory_parts tables
inventory_parts %>%
inner_join(parts, by = "part_num")
## # A tibble: 258,958 × 6
## inventory_id part_num color_id quantity name part_cat_id
## <dbl> <chr> <dbl> <dbl> <chr> <dbl>
## 1 21 3009 7 50 Brick 1 x 6 11
## 2 25 21019c00pat004pr1033 15 1 Legs and Hip… 61
## 3 25 24629pr0002 78 1 Minifig Head… 59
## 4 25 24634pr0001 5 1 Headwear Acc… 27
## 5 25 24782pr0001 5 1 Minifig Hipw… 27
## 6 25 88646 0 1 Tile Special… 15
## 7 25 973pr3314c01 5 1 Torso with 1… 60
## 8 26 14226c11 0 3 String with … 31
## 9 26 2340px2 15 1 Tail 4 x 1 x… 35
## 10 26 2340px3 15 1 Tail 4 x 1 x… 35
## # ℹ 258,948 more rows
6.1.3 Joining three or more tables
So far you’ve learned to join two tables together, but the same approach can join three or more. You can pipe any number of joins together, just like you can combine other dplyr verbs.
6.1.3.1 Joining three tables
You can string together multiple joins with inner_join
and the pipe (%>%
), both with which you are already very familiar!
We’ll now connect sets
, a table that tells us about each LEGO kit, with inventories
, a table that tells us the specific version of a given set, and finally to inventory_parts
, a table which tells us how many of each part is available in each LEGO kit.
So if you were building a Batman LEGO set, sets
would tell you the name of the set, inventories
would give you IDs for each of the versions of the set, and inventory_parts
would tell you how many of each part would be in each version.
Combine the inventories
table with the sets
table using the variable set_num
.
Next, join the inventory_parts
table to the table you created in the previous join by the inventory IDs. You will match id
with inventory_id
sets %>%
# Add inventories using an inner join
inner_join(inventories, by = "set_num") %>%
# Add inventory_parts using an inner join
inner_join(inventory_parts, by = c("id" = "inventory_id"))
## # A tibble: 258,958 × 9
## set_num name year theme_id id version part_num color_id quantity
## <chr> <chr> <dbl> <dbl> <dbl> <dbl> <chr> <dbl> <dbl>
## 1 700.3-1 Medium Gift … 1949 365 24197 1 bdoor01 2 2
## 2 700.3-1 Medium Gift … 1949 365 24197 1 bdoor01 15 1
## 3 700.3-1 Medium Gift … 1949 365 24197 1 bdoor01 4 1
## 4 700.3-1 Medium Gift … 1949 365 24197 1 bslot02 15 6
## 5 700.3-1 Medium Gift … 1949 365 24197 1 bslot02 2 6
## 6 700.3-1 Medium Gift … 1949 365 24197 1 bslot02 4 6
## 7 700.3-1 Medium Gift … 1949 365 24197 1 bslot02 1 6
## 8 700.3-1 Medium Gift … 1949 365 24197 1 bslot02 14 6
## 9 700.3-1 Medium Gift … 1949 365 24197 1 bslot02a 15 6
## 10 700.3-1 Medium Gift … 1949 365 24197 1 bslot02a 2 6
## # ℹ 258,948 more rows
6.1.4 What’s the most common color?
Now let’s join an additional table, colors
, which will tell us the color of each part in each set, so that we can answer the question, “what is the most common color of a LEGO piece?”
Inner join the colors
table using the color_id
column from the previous join and the id
column from colors
; use the suffixes "_set"
and "_color"
.
# Add an inner join for the colors table
sets %>%
inner_join(inventories, by = "set_num") %>%
inner_join(inventory_parts, by = c("id" = "inventory_id")) %>%
inner_join(colors, by = c("color_id" = "id"), suffix = c("_set", "_color" ))
## # A tibble: 258,958 × 11
## set_num name_set year theme_id id version part_num color_id quantity
## <chr> <chr> <dbl> <dbl> <dbl> <dbl> <chr> <dbl> <dbl>
## 1 700.3-1 Medium Gift … 1949 365 24197 1 bdoor01 2 2
## 2 700.3-1 Medium Gift … 1949 365 24197 1 bdoor01 15 1
## 3 700.3-1 Medium Gift … 1949 365 24197 1 bdoor01 4 1
## 4 700.3-1 Medium Gift … 1949 365 24197 1 bslot02 15 6
## 5 700.3-1 Medium Gift … 1949 365 24197 1 bslot02 2 6
## 6 700.3-1 Medium Gift … 1949 365 24197 1 bslot02 4 6
## 7 700.3-1 Medium Gift … 1949 365 24197 1 bslot02 1 6
## 8 700.3-1 Medium Gift … 1949 365 24197 1 bslot02 14 6
## 9 700.3-1 Medium Gift … 1949 365 24197 1 bslot02a 15 6
## 10 700.3-1 Medium Gift … 1949 365 24197 1 bslot02a 2 6
## # ℹ 258,948 more rows
## # ℹ 2 more variables: name_color <chr>, rgb <chr>
Count the name_color
column and sort the results so the most prominent colors appear first.
# Count the number of colors and sort
sets %>%
inner_join(inventories, by = "set_num") %>%
inner_join(inventory_parts, by = c("id" = "inventory_id")) %>%
inner_join(colors, by = c("color_id" = "id"), suffix = c("_set", "_color")) %>%
count(name_color, sort = TRUE)
## # A tibble: 134 × 2
## name_color n
## <chr> <int>
## 1 Black 48068
## 2 White 30105
## 3 Light Bluish Gray 26024
## 4 Red 21602
## 5 Dark Bluish Gray 19948
## 6 Yellow 17088
## 7 Blue 12980
## 8 Light Gray 8632
## 9 Reddish Brown 6960
## 10 Tan 6664
## # ℹ 124 more rows
6.2 Left and Right Joins
We need this to work with what they give us. You can run it at the start of this section
inventory_parts_joined <- sets %>%
inner_join(inventories, by = "set_num") %>%
inner_join(inventory_parts, by = c("id" = "inventory_id")) %>%
inner_join(colors, by = c("color_id" = "id"), suffix = c("_set", "_color")) %>%
select(set_num, part_num, color_id, quantity)
6.2.1 The left_join verb
An inner join keeps only observations that appear in both tables. But if you want to keep all the observations in one of the tables, you can use a different dplyr verb: left join.
6.2.1.1 Left joining two sets by part and color
In the video, you learned how to left join two LEGO sets.
Left join the star_destroyer
and millennium_falcon
tables on the part_num
and color_id
columns with the suffixes _falcon
and _star_destroyer
.
# Load in data sets given in data camp
millennium_falcon <- inventory_parts_joined %>%
filter(set_num == "7965-1")
star_destroyer <- inventory_parts_joined %>%
filter(set_num == "75190-1")
# Combine the star_destroyer and millennium_falcon tables
millennium_falcon %>%
left_join(star_destroyer, by = c("part_num", "color_id"), suffix = c("_falcon", "_star_destroyer"))
## # A tibble: 263 × 6
## set_num_falcon part_num color_id quantity_falcon set_num_star_destroyer
## <chr> <chr> <dbl> <dbl> <chr>
## 1 7965-1 12825 72 3 <NA>
## 2 7965-1 2412b 72 20 75190-1
## 3 7965-1 2412b 320 2 <NA>
## 4 7965-1 2419 71 1 <NA>
## 5 7965-1 2420 0 4 75190-1
## 6 7965-1 2420 71 1 <NA>
## 7 7965-1 2420 71 7 <NA>
## 8 7965-1 2431 72 2 <NA>
## 9 7965-1 2431 0 1 75190-1
## 10 7965-1 2431 19 2 <NA>
## # ℹ 253 more rows
## # ℹ 1 more variable: quantity_star_destroyer <dbl>
6.2.1.2 Left joining two sets by color
In the videos and the last exercise, you joined two sets based on their part and color. What if you joined the datasets by color alone?
Sum the quantity
column by color_id
in the Millennium Falcon dataset.
# Aggregate Millennium Falcon for the total quantity in each part
millennium_falcon_colors <- millennium_falcon %>%
group_by(color_id) %>%
summarize(total_quantity = sum(quantity))
Now, sum the quantity
column by color_id
in the Star Destroyer dataset.
# Aggregate Star Destroyer for the total quantity in each part
star_destroyer_colors <- star_destroyer %>%
group_by(color_id) %>%
summarize(total_quantity = sum(quantity))
Left join the two datasets, millennium_falcon_colors
and star_destroyer_colors
, using the color_id column
and the _falcon
and _star_destroyer suffixes
.
# Left join the Millennium Falcon colors to the Star Destroyer colors
millennium_falcon_colors %>%
left_join(star_destroyer_colors, by = "color_id", suffix=c("_falcon", "_star_destroyer"))
## # A tibble: 21 × 3
## color_id total_quantity_falcon total_quantity_star_destroyer
## <dbl> <dbl> <dbl>
## 1 0 201 336
## 2 1 15 23
## 3 4 17 53
## 4 14 3 4
## 5 15 15 17
## 6 19 95 12
## 7 28 3 16
## 8 33 5 NA
## 9 36 1 14
## 10 41 6 15
## # ℹ 11 more rows
6.2.1.3 Finding an observation that doesn’t have a match
Left joins are really great for testing your assumptions about a data set and ensuring your data has integrity.
For example, the inventories
table has a version
column, for when a LEGO kit gets some kind of change or upgrade. It would be fair to assume that all sets
(which joins well with inventories
) would have at least a version 1. But let’s test this assumption out in the following exercise.
Use a left_join
to join together sets
and inventory_version_1
using their common column (set_num
).
filter
for where the version
column is NA
using is.na
using filter(is.na(version))
inventory_version_1 <- inventories %>%
filter(version == 1)
# Join versions to sets
sets %>%
left_join(inventory_version_1, by = "set_num") %>%
# Filter for where version is na
filter(is.na(version))
## # A tibble: 1 × 6
## set_num name year theme_id id version
## <chr> <chr> <dbl> <dbl> <dbl> <dbl>
## 1 40198-1 Ludo game 2018 598 NA NA
6.2.2 The right_join verb
In the last lesson, you learned about the left join verb. It might not surprise you to learn that there’s also a right join. Just as left joins keep all the observations from the first (or “left”) table, whether or not they appear in the second (or “right”) table, a right join keeps all the observations in the second (or “right”) table, whether or not they appear in the first table.
6.2.2.1 Counting part colors
Sometimes you’ll want to do some processing before you do a join, and prioritize keeping the second (right) table’s rows instead. In this case, a right join is for you.
In this exercise, we’ll count the part_cat_id
from parts
, before using a right_join
to join with part_categories
. The reason we do this is because we don’t only want to know the count of part_cat_id
in parts
, but we also want to know if there are any part_cat_ids
not present in parts
.
Use the count
verb to count each part_cat_id
in the parts table.
Use a right_join
to join part_categories
. You’ll need to use the part_cat_id
from the count
and the id
column from part_categories
.
parts %>%
# Count the part_cat_id
count(part_cat_id) %>%
# Right join part_categories
right_join(part_categories, by = c("part_cat_id" = "id"))
## # A tibble: 64 × 3
## part_cat_id n name
## <dbl> <int> <chr>
## 1 1 135 Baseplates
## 2 3 303 Bricks Sloped
## 3 4 1900 Duplo, Quatro and Primo
## 4 5 107 Bricks Special
## 5 6 128 Bricks Wedged
## 6 7 97 Containers
## 7 8 24 Technic Bricks
## 8 9 167 Plates Special
## 9 11 490 Bricks
## 10 12 85 Technic Connectors
## # ℹ 54 more rows
filter
for where the column n
is NA.
parts %>%
count(part_cat_id) %>%
right_join(part_categories, by = c("part_cat_id" = "id")) %>%
# Filter for NA
filter(is.na(n))
## # A tibble: 1 × 3
## part_cat_id n name
## <dbl> <int> <chr>
## 1 66 NA Modulex
6.2.2.2 Cleaning up your count
In both left and right joins, there is the opportunity for there to be NA values in the resulting table. Fortunately, the replace_na
function can turn those NAs into meaningful values.
In the last exercise, we saw that the n column had NAs after the right_join
. Let’s use the replace_na
column, which takes a list of column names and the values with which NAs should be replaced, to clean up our table.
Use replace_na
to replace NAs in the n
column with the value 0
. (hint: you must use list()
inside the replace_na
function)
parts %>%
count(part_cat_id) %>%
right_join(part_categories, by = c("part_cat_id" = "id")) %>%
# Use replace_na to replace missing values in the n column
replace_na(list(n = 0))
## # A tibble: 64 × 3
## part_cat_id n name
## <dbl> <int> <chr>
## 1 1 135 Baseplates
## 2 3 303 Bricks Sloped
## 3 4 1900 Duplo, Quatro and Primo
## 4 5 107 Bricks Special
## 5 6 128 Bricks Wedged
## 6 7 97 Containers
## 7 8 24 Technic Bricks
## 8 9 167 Plates Special
## 9 11 490 Bricks
## 10 12 85 Technic Connectors
## # ℹ 54 more rows
6.2.3 Joining tables to themselves
You can also join a table to itself, by matching each theme to its parents
6.2.4 Joining themes to their children
Tables can be joined to themselves!
In the themes
table, which is available for you to inspect in the console, you’ll notice there is both an id
column and a parent_id
column. Keeping that in mind, you can join the themes
table to itself to determine the parent-child relationships that exist for different themes.
In the videos, you saw themes joined to their own parents. In this exercise, you’ll try a similar approach of joining themes to their own children, which is similar but reversed. Let’s try this out to discover what children the theme “Harry Potter
” has.
Inner join themes
to their own children, resulting in the suffixes "_parent"
and "_child"
, respectively.
Filter this table to find the children of the "Harry Potter"
theme.
themes %>%
# Inner join the themes table
inner_join(themes, by = c("id" = "parent_id"), suffix = c("_parent", "_child")) %>%
# Filter for the "Harry Potter" parent name
filter(name_parent == "Harry Potter")
## # A tibble: 6 × 5
## id name_parent parent_id id_child name_child
## <dbl> <chr> <dbl> <dbl> <chr>
## 1 246 Harry Potter NA 247 Chamber of Secrets
## 2 246 Harry Potter NA 248 Goblet of Fire
## 3 246 Harry Potter NA 249 Order of the Phoenix
## 4 246 Harry Potter NA 250 Prisoner of Azkaban
## 5 246 Harry Potter NA 251 Sorcerer's Stone
## 6 246 Harry Potter NA 667 Fantastic Beasts
6.2.4.1 Joining themes to their grandchildren
We can go a step further than looking at themes and their children. Some themes actually have grandchildren: their children’s children.
Here, we can inner join themes
to a filtered version of itself again to establish a connection between our last join’s children and their children.
Be sure to use the suffixes "_parent"
and "_grandchild"
so the columns in the resulting table are clear.
Update the by
argument to specify the correct columns to join on. If you’re unsure of what columns to join on, it might help to look at the result of the first join to get a feel for it.
# Join themes to itself again to find the grandchild relationships
themes %>%
inner_join(themes, by = c("id" = "parent_id"), suffix = c("_parent", "_child")) %>%
inner_join(themes, by = c("id_child" = "parent_id"), suffix = c("_parent", "_grandchild"))
## # A tibble: 158 × 7
## id_parent name_parent parent_id id_child name_child id_grandchild name
## <dbl> <chr> <dbl> <dbl> <chr> <dbl> <chr>
## 1 1 Technic NA 5 Model 6 Airport
## 2 1 Technic NA 5 Model 7 Constructi…
## 3 1 Technic NA 5 Model 8 Farm
## 4 1 Technic NA 5 Model 9 Fire
## 5 1 Technic NA 5 Model 10 Harbor
## 6 1 Technic NA 5 Model 11 Off-Road
## 7 1 Technic NA 5 Model 12 Race
## 8 1 Technic NA 5 Model 13 Riding Cyc…
## 9 1 Technic NA 5 Model 14 Robot
## 10 1 Technic NA 5 Model 15 Traffic
## # ℹ 148 more rows
6.2.4.2 Left joining a table to itself
So far, you’ve been inner joining a table to itself in order to find the children of themes like "Harry Potter"
or "The Lord of the Rings"
.
But some themes might not have any children at all, which means they won’t be included in the inner join. As you’ve learned in this chapter, you can identify those with a left_join
and a filter()
.
Left join the themes table to its own children, with the suffixes _parent
and _child
respectively.
Filter the result of the join to find themes that have no children.
themes %>%
# Left join the themes table to its own children
left_join(themes, by = c("id" = "parent_id"), suffix = c("_parent", "_child")) %>%
# Filter for themes that have no child themes
filter(is.na(name_child))
## # A tibble: 586 × 5
## id name_parent parent_id id_child name_child
## <dbl> <chr> <dbl> <dbl> <chr>
## 1 2 Arctic Technic 1 NA <NA>
## 2 3 Competition 1 NA <NA>
## 3 4 Expert Builder 1 NA <NA>
## 4 6 Airport 5 NA <NA>
## 5 7 Construction 5 NA <NA>
## 6 8 Farm 5 NA <NA>
## 7 9 Fire 5 NA <NA>
## 8 10 Harbor 5 NA <NA>
## 9 11 Off-Road 5 NA <NA>
## 10 12 Race 5 NA <NA>
## # ℹ 576 more rows
6.3 Full, Semi, and Anti Joins
6.3.1 The full_join verb
What if instead of keeping all the observations in the left or the right tables, you wanted to keep all observations in both tables, whether or not they matched to each other? In this lesson: you’ll learn another of dplyr’s joining verbs: full join.
6.3.1.1 Differences between Batman and Star Wars
In the video, you compared two sets. Now, you’ll compare two themes, each of which is made up of many sets.
In order to join in the themes, you’ll first need to combine the inventory_parts_joined
and sets
tables.
Then, combine the first join with the themes
table, using the suffix argument to clarify which table each name came from ("_set"
or "_theme"
).
# create the data set used in data camp
inventory_parts_joined <- inventories %>%
inner_join(inventory_parts, by = c("id" = "inventory_id")) %>%
arrange(desc(quantity)) %>%
select(-id, -version)
# Start with inventory_parts_joined table
inventory_parts_joined %>%
# Combine with the sets table
inner_join(sets, by = "set_num") %>%
# Combine with the themes table
inner_join(themes, by = c("theme_id" = "id"), suffix = c("_set", "_theme"))
## # A tibble: 258,958 × 9
## set_num part_num color_id quantity name_set year theme_id name_theme
## <chr> <chr> <dbl> <dbl> <chr> <dbl> <dbl> <chr>
## 1 40179-1 3024 72 900 Personalised M… 2016 277 Mosaic
## 2 40179-1 3024 15 900 Personalised M… 2016 277 Mosaic
## 3 40179-1 3024 0 900 Personalised M… 2016 277 Mosaic
## 4 40179-1 3024 71 900 Personalised M… 2016 277 Mosaic
## 5 40179-1 3024 14 900 Personalised M… 2016 277 Mosaic
## 6 k34434-1 3024 15 810 Lego Mosaic Ti… 2003 277 Mosaic
## 7 21010-1 3023 320 771 Robie House 2011 252 Architect…
## 8 k34431-1 3024 0 720 Lego Mosaic Cat 2003 277 Mosaic
## 9 42083-1 2780 0 684 Bugatti Chiron 2018 5 Model
## 10 k34434-1 3024 0 540 Lego Mosaic Ti… 2003 277 Mosaic
## # ℹ 258,948 more rows
## # ℹ 1 more variable: parent_id <dbl>
6.3.1.2 Aggregating each theme
Previously, you combined tables to compare themes. Before doing this comparison, you’ll want to aggregate the data to learn more about the pieces that are a part of each theme, as well as the colors of those pieces.
Count the part number and color id for the parts in Batman and Star Wars, weighted by quantity.
#create datasets used in data camp
inventory_sets_themes <- inventory_parts_joined %>%
inner_join(sets, by = "set_num") %>%
inner_join(themes, by = c("theme_id" = "id"), suffix = c("_set", "_theme"))
batman <- inventory_sets_themes %>%
filter(name_theme == "Batman")
star_wars <- inventory_sets_themes %>%
filter(name_theme == "Star Wars")
# Count the part number and color id, weight by quantity
batman %>%
count(part_num, color_id, wt = quantity)
## # A tibble: 2,071 × 3
## part_num color_id n
## <chr> <dbl> <dbl>
## 1 10113 0 11
## 2 10113 272 1
## 3 10113 320 1
## 4 10183 57 1
## 5 10190 0 2
## 6 10201 0 1
## 7 10201 4 3
## 8 10201 14 1
## 9 10201 15 6
## 10 10201 71 4
## # ℹ 2,061 more rows
## # A tibble: 2,413 × 3
## part_num color_id n
## <chr> <dbl> <dbl>
## 1 10169 4 1
## 2 10197 0 2
## 3 10197 72 3
## 4 10201 0 21
## 5 10201 71 5
## 6 10247 0 9
## 7 10247 71 16
## 8 10247 72 12
## 9 10884 28 1
## 10 10928 72 6
## # ℹ 2,403 more rows
6.3.1.3 Full joining Batman and Star Wars LEGO parts
Now that you’ve got separate tables for the pieces in the batman and star_wars themes, you’ll want to be able to combine them to see any similarities or differences between the two themes.
Combine the star_wars_parts
table with the batman_parts
table; use the suffix argument to include the "_batman"
and "_star_wars"
suffixes.
Replace all the NA values in the n_batman
and n_star_wars
columns with 0s.
# create data sets used in data camp
batman_parts <- batman %>%
count(part_num, color_id, wt = quantity)
star_wars_parts <- star_wars %>%
count(part_num, color_id, wt = quantity)
batman_parts %>%
# Combine the star_wars_parts table
full_join(star_wars_parts, by = c("part_num", "color_id"), suffix = c("_batman", "_star_wars")) %>%
# Replace NAs with 0s in the n_batman and n_star_wars columns
replace_na(list(n_batman = 0, n_star_wars = 0))
## # A tibble: 3,628 × 4
## part_num color_id n_batman n_star_wars
## <chr> <dbl> <dbl> <dbl>
## 1 10113 0 11 0
## 2 10113 272 1 0
## 3 10113 320 1 0
## 4 10183 57 1 0
## 5 10190 0 2 0
## 6 10201 0 1 21
## 7 10201 4 3 0
## 8 10201 14 1 0
## 9 10201 15 6 0
## 10 10201 71 4 5
## # ℹ 3,618 more rows
6.3.1.4 Comparing Batman and Star Wars LEGO parts
The table you created in the last exercise includes the part number of each piece, the color id, and the number of each piece in the Star Wars and Batman themes. However, we have more information about each of these parts that we can gain by combining this table with some of the information we have in other tables. Before we compare the themes, let’s ensure that we have enough information to make our findings more interpretable.
Sort the number of star wars pieces in the parts_joined
table in descending order.
Inner join the colors
table to the parts_joined
table.
Combine the parts
table to the previous join using an inner join; add "_color"
and "_part"
suffixes to specify whether or not the information came from the colors
table or the parts
table.
#create data sets used in data camp
parts_joined <- batman_parts %>%
full_join(star_wars_parts, by = c("part_num", "color_id"), suffix = c("_batman", "_star_wars")) %>%
replace_na(list(n_batman = 0, n_star_wars = 0))
parts_joined %>%
# Sort the number of star wars pieces in descending order
arrange(desc(n_star_wars)) %>%
# Join the colors table to the parts_joined table
inner_join(colors, by = c("color_id" = "id")) %>%
# Join the parts table to the previous join
inner_join(parts, by = "part_num", suffix = c("_color", "_part"))
## # A tibble: 3,628 × 8
## part_num color_id n_batman n_star_wars name_color rgb name_part part_cat_id
## <chr> <dbl> <dbl> <dbl> <chr> <chr> <chr> <dbl>
## 1 2780 0 104 392 Black #051… Technic … 53
## 2 32062 0 1 141 Black #051… Technic … 46
## 3 4274 1 56 118 Blue #005… Technic … 53
## 4 6141 36 11 117 Trans-Red #C91… Plate Ro… 21
## 5 3023 71 10 106 Light Blu… #A0A… Plate 1 … 14
## 6 6558 1 30 106 Blue #005… Technic … 53
## 7 43093 1 44 99 Blue #005… Technic … 53
## 8 3022 72 14 95 Dark Blui… #6C6… Plate 2 … 14
## 9 2357 19 0 84 Tan #E4C… Brick 2 … 11
## 10 6141 179 90 81 Flat Silv… #898… Plate Ro… 21
## # ℹ 3,618 more rows
6.3.2 The semi_join and anti_join verbs
A filtering join keeps or removes observations from the first table, but it doesn’t add new variables. The two filtering verbs you’ll be learning are semi join and anti join. A semi join asks the question: what observations in X are also in Y? And an anti join asks the question: what observations in X are not in Y?
6.3.2.1 Something within one set but not another
In the videos, you learned how to filter using the semi- and anti join verbs to answer questions you have about your data. Let’s focus on the batwing
dataset, and use our skills to determine which parts are in both the batwing
and batmobile
sets, and which sets are in one, but not the other. While answering these questions, we’ll also be determining whether or not the parts we’re looking at in both sets also have the same color in common.
Filter the batwing
set for parts that are also in the batmobile
, whether or not they have the same color.
Filter the batwing
set for parts that aren’t also in the batmobile
, whether or not they have the same color.
#load in data sets used in datacamp
batmobile <- inventory_parts_joined %>%
filter(set_num == "7784-1") %>%
select(-set_num)
batwing <- inventory_parts_joined %>%
filter(set_num == "70916-1") %>%
select(-set_num)
# Filter the batwing set for parts that are also in the batmobile set
batwing %>%
semi_join(batmobile, by = "part_num")
## # A tibble: 126 × 3
## part_num color_id quantity
## <chr> <dbl> <dbl>
## 1 3023 0 22
## 2 3024 0 22
## 3 3623 0 20
## 4 2780 0 17
## 5 3666 0 16
## 6 3710 0 14
## 7 6141 4 12
## 8 2412b 71 10
## 9 6141 72 10
## 10 6558 1 9
## # ℹ 116 more rows
# Filter the batwing set for parts that aren't in the batmobile set
batwing %>%
anti_join(batmobile, by = "part_num")
## # A tibble: 183 × 3
## part_num color_id quantity
## <chr> <dbl> <dbl>
## 1 11477 0 18
## 2 99207 71 18
## 3 22385 0 14
## 4 99563 0 13
## 5 10247 72 12
## 6 2877 72 12
## 7 61409 72 12
## 8 11153 0 10
## 9 98138 46 10
## 10 2419 72 9
## # ℹ 173 more rows
6.3.2.2 What colors are included in at least one set?
Besides comparing two sets directly, you could also use a filtering join like semi_join
to find out which colors ever appear in any inventory part. Some of the colors could be optional, meaning they aren’t included in any sets.
Use the inventory_parts
table to find the colors that are included in at least one set.
# Use inventory_parts to find colors included in at least one set
colors %>%
semi_join(inventory_parts, by = c("id" = "color_id"))
## # A tibble: 134 × 3
## id name rgb
## <dbl> <chr> <chr>
## 1 -1 [Unknown] #0033B2
## 2 0 Black #05131D
## 3 1 Blue #0055BF
## 4 2 Green #237841
## 5 3 Dark Turquoise #008F9B
## 6 4 Red #C91A09
## 7 5 Dark Pink #C870A0
## 8 6 Brown #583927
## 9 7 Light Gray #9BA19D
## 10 8 Dark Gray #6D6E5C
## # ℹ 124 more rows
6.3.2.3 Which set is missing version 1?
Each set included in the LEGO data has an associated version number. We want to understand the version we are looking at to learn more about the parts that are included. Before doing that, we should confirm that there aren’t any sets that are missing a particular version.
Let’s start by looking at the first version of each set to see if there are any sets that don’t include a first version.
Use filter()
to extract version 1
from the inventories
table; save the filter to version_1_inventories
.
Use anti_join
to combine version_1_inventories
with sets
to determine which set is missing a version 1.
# Use filter() to extract version 1
version_1_inventories <- inventories %>%
filter(version == 1)
# Use anti_join() to find which set is missing a version 1
sets %>%
anti_join(version_1_inventories, by = "set_num")
## # A tibble: 1 × 4
## set_num name year theme_id
## <chr> <chr> <dbl> <dbl>
## 1 40198-1 Ludo game 2018 598
6.3.3 Visualizing set differences
6.3.3.1 Aggregating sets to look at their differences
To compare two individual sets, and the kinds of LEGO pieces that comprise them, we’ll need to aggregate the data into separate themes. Additionally, as we saw in the video, we’ll want to add a column so that we can understand the fractions of specific pieces that are part of each set, rather than looking at the numbers of pieces alone.
Add a filter for the "Batman"
theme to create the batman_colors
object.
Add a fraction
column to batman_colors
that displays the total divided by the sum of the total.
Repeat the steps to filter and aggregate the "Star Wars"
set data to create the star_wars_colors
object.
Add a fraction column to star_wars_colors
to display the fraction of the total.
#load in dataset used in data camp
inventory_parts_themes <- inventories %>%
inner_join(inventory_parts, by = c("id" = "inventory_id")) %>%
arrange(desc(quantity)) %>%
select(-id, -version) %>%
inner_join(sets, by = "set_num") %>%
inner_join(themes, by = c("theme_id" = "id"), suffix = c("_set", "_theme"))
batman_colors <- inventory_parts_themes %>%
# Filter the inventory_parts_themes table for the Batman theme
filter(name_theme == "Batman") %>%
group_by(color_id) %>%
summarize(total = sum(quantity)) %>%
# Add a fraction column of the total divided by the sum of the total
mutate(fraction = total / sum(total))
# Filter and aggregate the Star Wars set data; add a fraction column
star_wars_colors <- inventory_parts_themes %>%
filter(name_theme == "Star Wars") %>%
group_by(color_id) %>%
summarize(total = sum(quantity)) %>%
mutate(fraction = total / sum(total))
6.3.3.2 Combining sets
Join the batman_colors
and star_wars_colors
tables; be sure to include all
observations from both tables.
Replace the NAs in the total_batman
and total_star_wars
columns.
batman_colors %>%
# Join the Batman and Star Wars colors
full_join(star_wars_colors, by = "color_id", suffix = c("_batman", "_star_wars")) %>%
# Replace NAs in the total_batman and total_star_wars columns
replace_na(list(total_batman = 0, total_star_wars = 0)) %>%
inner_join(colors, by = c("color_id" = "id"))
## # A tibble: 63 × 7
## color_id total_batman fraction_batman total_star_wars fraction_star_wars
## <dbl> <dbl> <dbl> <dbl> <dbl>
## 1 0 2807 0.296 3258 0.207
## 2 1 243 0.0256 410 0.0261
## 3 2 158 0.0167 36 0.00229
## 4 4 529 0.0558 434 0.0276
## 5 5 1 0.000105 0 NA
## 6 10 13 0.00137 6 0.000382
## 7 14 426 0.0449 207 0.0132
## 8 15 404 0.0426 1771 0.113
## 9 19 142 0.0150 1012 0.0644
## 10 25 36 0.00380 36 0.00229
## # ℹ 53 more rows
## # ℹ 2 more variables: name <chr>, rgb <chr>
Add a difference
column which is the difference between fraction_batman
and fraction_star_wars
, and a total
column, which is the sum of total_batman
and total_star_wars
.
Add a filter
to select observations where total
is at least 200.
colors_joined <- batman_colors %>%
full_join(star_wars_colors, by = "color_id", suffix = c("_batman", "_star_wars")) %>%
replace_na(list(total_batman = 0, total_star_wars = 0)) %>%
inner_join(colors, by = c("color_id" = "id")) %>%
# Create the difference and total columns
mutate(difference = fraction_batman - fraction_star_wars,
total = total_batman + total_star_wars) %>%
# Filter for totals greater than 200
filter(total >= 200)
6.3.3.3 Visualizing the difference: Batman and Star Wars
If you want to make the graph in the last exercise of this DC chapter, you’ll need to adjust the color names in the data and create the color pallet. It uses colors_joined
, so once you have gotten to where you create colors_joined
, change put this code chunk after where you create colors_joined
and change the code chunk option to eval=TRUE
.
# For some reason I got one color with a difference of NA...
# you don't have to drop it, but you avoid an error if you do.
# Even better is figuring out how to avoid the NA in the first place...
# You also need to arrange the data by difference (that's how it is in the graph)
colors_joined <- colors_joined %>% arrange(difference) %>% filter(!is.na(difference))
# These two lines get the color names to display in order of difference.
# There are other ways (they mention the "forcats" package in the video),
# but like many things, I googled it and I found a solution tat I adapted to this and it worked
colors_joined$name <- as.character(colors_joined$name)
colors_joined$name <- factor(colors_joined$name, levels=colors_joined$name)
# Create the color palette itself, which is just the colors and their names
color_palette_df <- colors %>%
semi_join(colors_joined, by = c("id" = "color_id")) %>%
select(-id)
color_palette <- color_palette_df$rgb
names(color_palette) <- color_palette_df$name
Create a bar plot using the colors_joined
table to display the most prominent colors in the Batman and Star Wars themes, with the bars colored by their name
.
# Create a bar plot using colors_joined and the name and difference columns
ggplot(colors_joined, aes(x=name, y=difference, fill = name)) +
geom_col() +
coord_flip() +
scale_fill_manual(values = color_palette, guide = "none") +
labs(y = "Difference: Batman - Star Wars")
6.4 Case Study: Joins on Stack Overflow Data
You’ve also seen how they can be applied to combine data across a number of tables describing LEGO toys. For this last chapter, you’re going to apply everything you’ve learned to a different dataset, to see how these joining verbs are useful in a variety of circumstances.
6.4.1 Stack Overflow questions
The questions table contains each of the almost 300,000 Stack Oveflow questions that are tagged with R, along with the date they were asked and their score. A positive score means people upvoted the question, a negative means they downvoted it.
6.4.2 Joining questions and answers
The answers table has an id, creation date, and score, just like the questions table, but it also has a question ID, which links to the questions table. This means we could join them based on those columns.
6.4.2.1 Finding gaps between questions and answers
Now we’ll join together questions
with answers
so we can measure the time between questions and answers.
Make sure to explore the tables and columns in the console before starting the exercise. Can you tell how are questions identified in the questions table? How can you identify which answer corresponds to which question
using the answers
table?
Use an inner join to combine the questions
and answers
tables using the suffixes "_question"
and "_answer"
, respectively.
Subtract creation_date_question
from creation_date_answer
within the as.integer()
function to create the gap
column.
questions %>%
# Inner join questions and answers with proper suffixes
inner_join(answers, by = c("id" = "question_id"), suffix = c("_question", "_answer")) %>%
# Subtract creation_date_question from creation_date_answer to create gap
mutate(gap = as.integer(creation_date_question - creation_date_answer))
## # A tibble: 380,643 × 7
## id creation_date_question score_question id_answer creation_date_answer
## <int> <date> <int> <int> <date>
## 1 22557677 2014-03-21 1 22560670 2014-03-21
## 2 22557707 2014-03-21 2 22558516 2014-03-21
## 3 22557707 2014-03-21 2 22558726 2014-03-21
## 4 22558084 2014-03-21 2 22558085 2014-03-21
## 5 22558084 2014-03-21 2 22606545 2014-03-24
## 6 22558084 2014-03-21 2 22610396 2014-03-24
## 7 22558084 2014-03-21 2 34374729 2015-12-19
## 8 22558395 2014-03-21 2 22559327 2014-03-21
## 9 22558395 2014-03-21 2 22560102 2014-03-21
## 10 22558395 2014-03-21 2 22560288 2014-03-21
## # ℹ 380,633 more rows
## # ℹ 2 more variables: score_answer <int>, gap <int>
6.4.2.2 Joining question and answer counts
We can also determine how many questions actually yield answers. If we count the number of answers for each question, we can then join the answers counts with the questions
table.
Count and sort the question_id
column in the answers
table to create the answer_counts` table.
Join the questions
table with the answer_counts
table and include all observations from the questions
table.
Replace the NA values in the n
column with 0s.
# Count and sort the question id column in the answers table
answer_counts <- answers %>%
count(question_id, sort = TRUE)
# Combine the answer_counts and questions tables
questions %>%
left_join(answer_counts, by = c("id" = "question_id")) %>%
# Replace the NAs in the n column
replace_na(list(n = 0))
## # A tibble: 294,735 × 4
## id creation_date score n
## <int> <date> <int> <int>
## 1 22557677 2014-03-21 1 1
## 2 22557707 2014-03-21 2 2
## 3 22558084 2014-03-21 2 4
## 4 22558395 2014-03-21 2 3
## 5 22558613 2014-03-21 0 1
## 6 22558677 2014-03-21 2 2
## 7 22558887 2014-03-21 8 1
## 8 22559180 2014-03-21 1 1
## 9 22559312 2014-03-21 0 1
## 10 22559322 2014-03-21 2 5
## # ℹ 294,725 more rows
6.4.2.4 Average answers by question
Some of the important variables from this table include: n
, the number of answers for each question, and tag_name
, the name of each tag associated with each question.
Let’s use some of our favorite dplyr verbs to find out how many answers each question gets on average.
Aggregate the tagged_answers
table by tag_name
.
Summarize tagged_answers
to get the count of questions
and the average_answers
.
Sort the resulting questions
column in descending order.
tagged_answers %>%
# Aggregate by tag_name
group_by(tag_name) %>%
# Summarize questions and average_answers
summarize(questions = n(),
average_answers = mean(n)) %>%
# Sort the questions in descending order
arrange(desc(questions))
## # A tibble: 7,840 × 3
## tag_name questions average_answers
## <chr> <int> <dbl>
## 1 ggplot2 28228 1.15
## 2 dataframe 18874 1.67
## 3 shiny 14219 0.921
## 4 dplyr 14039 1.55
## 5 plot 11315 1.23
## 6 data.table 8809 1.47
## 7 matrix 6205 1.45
## 8 loops 5149 1.39
## 9 regex 4912 1.91
## 10 function 4892 1.30
## # ℹ 7,830 more rows